From Data Warehouses to Data Lakehouses
The landscape of data storage and processing has undergone a remarkable transformation over the past few decades, driven by the exponential growth of data and the need for more advanced analytical capabilities. Initially, data warehouses emerged as the go-to solution for storing and managing structured data from various sources. These centralized repositories enabled businesses to perform efficient querying, reporting, and analysis, providing critical insights that informed strategic decision-making. However, the rigid architecture of data warehouses posed challenges in adapting to the increasing volume and variety of data, particularly unstructured data.
In response to these limitations, data lakes emerged in the early 2010s, offering a more flexible and scalable approach to data storage. Unlike data warehouses, data lakes could handle vast amounts of raw data in its native format, supporting both structured and unstructured data. This flexibility allowed organizations to leverage big data for advanced analytics and real-time processing. Despite their advantages, data lakes often faced issues related to data governance and quality, leading to the advent of data lakehouses. Combining the strengths of both data warehouses and data lakes, data lakehouses provide a unified platform that ensures efficient data management, governance, and performance optimization, enabling organizations to make more informed decisions and drive innovation.
Understanding Data Warehouses: Pros and Cons
Data warehouses have been a cornerstone of business intelligence since their inception in the 1980s. Designed to consolidate and store large volumes of structured data from various sources, data warehouses enable efficient querying, reporting, and analysis. Their architecture is optimized for read-heavy operations, making them ideal for historical data analysis and generating business insights. With robust data integration capabilities, data warehouses ensure data consistency and reliability, which are critical for accurate decision-making processes.
However, data warehouses are not without their limitations. Their rigid schema design and reliance on structured data make them less adaptable to the growing influx of unstructured data. Additionally, the process of transforming and loading data into a warehouse can be time-consuming and resource-intensive. Scaling a data warehouse to accommodate large data volumes can also be costly and complex. Despite these challenges, data warehouses remain a vital tool for businesses that require consistent and reliable access to historical data for analytical purposes.
Pros:
- Optimized for read-heavy operations
- Ensures data consistency and reliability
- Ideal for historical data analysis
- Strong data integration capabilities
Cons:
- Rigid schema design
- Limited adaptability to unstructured data
- Time-consuming data transformation and loading processes
- Costly and complex to scale for large data volumes
Exploring Data Lakes: Advantages and Drawbacks
Data lakes emerged as a solution to the limitations of traditional data warehouses, particularly in handling the explosion of unstructured data from diverse sources such as social media, IoT devices, and logs. Unlike data warehouses, which require data to be structured and transformed before storage, data lakes can store raw data in its native format. This flexibility enables organizations to capture and analyze a wide variety of data types, supporting advanced analytics, machine learning, and real-time processing. Data lakes provide scalability, accommodating vast amounts of data without the need for a predefined schema, making them ideal for big data environments.
Despite their numerous benefits, data lakes also come with certain challenges. The lack of inherent data governance and management can lead to issues with data quality, often resulting in what is termed as a "data swamp" if not properly managed. Without proper structure and organization, retrieving specific data can be time-consuming and complex. Additionally, the schema-on-read approach, while flexible, can complicate data consistency and reliability.
However, with appropriate data governance and management strategies, data lakes can offer a powerful solution for modern data storage and processing needs.
Pros:
- Handles both structured and unstructured data
- Scalable for large volumes of data
- Supports advanced analytics and real-time processing
- Stores raw data in its native format
Cons:
- Potential for poor data quality and "data swamps"
- Can be complex and time-consuming to retrieve specific data
- Schema-on-read approach complicates data consistency
- Requires robust data governance and management strategies
Data Lakehouses: The Latest Evolution in Data Management
Data lakehouses represent the latest innovation in data storage and processing, combining the strengths of both data warehouses and data lakes while addressing their respective limitations. A data lakehouse provides a unified architecture that supports the storage of structured, semi-structured, and unstructured data, offering the scalability and flexibility of a data lake with the data management, governance, and performance optimization features of a data warehouse. This hybrid approach allows organizations to conduct complex analytics, machine learning, and real-time data processing more efficiently and effectively.
The integration of the best features from data warehouses and data lakes makes data lakehouses a powerful solution for modern data challenges. They facilitate better data governance, ensuring data quality and consistency, while still allowing for the flexible storage and processing of diverse data types. Additionally, data lakehouses support schema-on-read and schema-on-write capabilities, making data management more versatile and robust.
However, the complexity of implementing and managing a data lakehouse can be higher than traditional systems, and the technology is still evolving, which may present integration challenges for some organizations.
Pros:
- Combines strengths of data warehouses and data lakes
- Supports structured, semi-structured, and unstructured data
- Enhanced data governance and quality control
- Facilitates advanced analytics, machine learning, and real-time processing
Cons:
- More complex to implement and manage
- Technology is still evolving
- Potential integration challenges
- May require significant investment in infrastructure and expertise
Embrace the Future of Data Management
Navigating the evolution from data warehouses to data lakes and data lakehouses is crucial for staying ahead in today's data-driven world.
Avantia offers expert guidance to help you seamlessly transition through these stages, optimizing your data management strategy and harnessing the full potential of your data assets. Don’t get left behind—contact us today to revolutionize your data infrastructure and drive your business forward with cutting-edge solutions tailored to your needs.
Let's Partner
Avantia will work with your team to develop a data approach that fits your company and its needs.
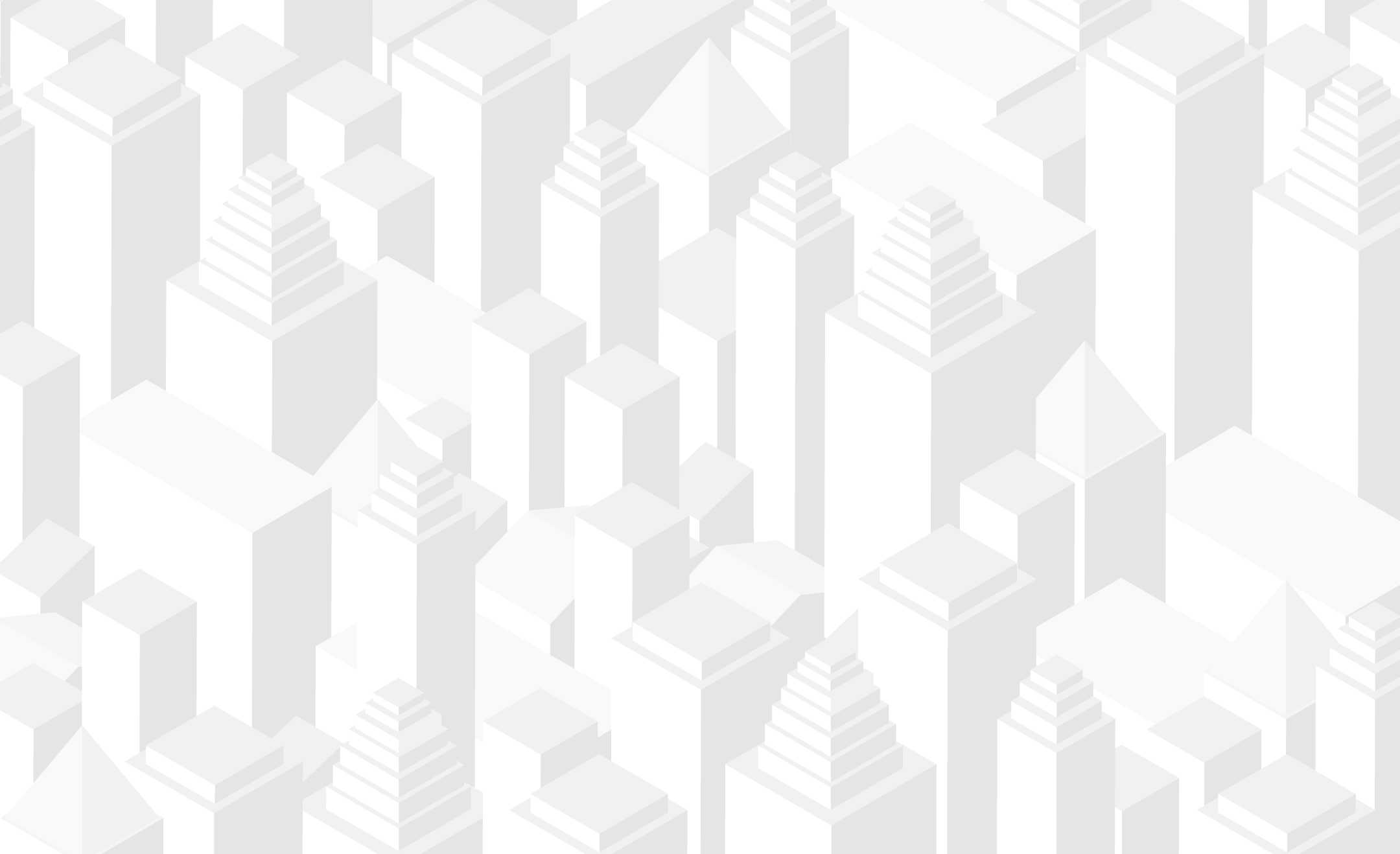